How Machine Learning, Classification Models Impact Marketing Ethics
Machine learning classification needs accurate data to avoid bad prediction that places marketing efforts in ethical peril. Here's an overview to help explain how data can brands at risk.
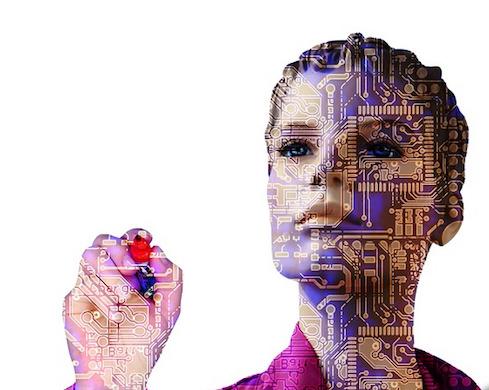
People seek convenience in their experiences with brands. Brands have begun to use machine learning classification to know who, where, and when to direct resources to provide that convenience.
But in relying on algorithms to provide customer convenience, managers must understand classification to protect brands from making unethical societal choices when delivering outcomes to customers.
It's not new for businesses to be proactive in an effort to influence. History is filled with intriguing stories of worthy trials such as constructing homes near plants for workers, to failed efforts, too, such as those that led to the 2009 global financial crisis. When businesses use technology to influence, an important question arises. What qualities become associated with algorithms? It starts with how data is classified.
Classification algorithms define rules to place observational data into a category or group. Various classification algorithms based on precise statistics are obtained through programming. Models such as regressions, Latent Dirichlet Allocation (LDA), or cluster analysis, can be recreated using Python, or R Programming.
The ethical challenge lies in creating the data for algorithm models. Modeling includes training datasets to train a given model while a validation dataset is used to choose the algorithm that accurately represents the environment.
Training datasets are increasingly incorporating real-world conditions.
For example, big data often includes data created from images. Digital images that were once useful as website elements can now be mapped out against location coordinates or aid in facial recognition. These associations can provide scientific value in the form of environmental impact, such as image recognition being used to map global warming impact on the coral reef. However, the variety of data types can also lead to models that incorporate societal risks or historical cultural bias associated with the data used in training.
Social concern about algorithm decisions comes as predictive models begin to incorporate data linked to historical social legacy. Thus the standards for data accuracy now must account for avoiding social and political mistakes that carry significant consequences. That is extremely important when a model is learning from the data provided, particularly when the learning is unsupervised.
For example, look at what happened to Amazon Prime. Bloomberg reported how Amazon Prime Same Day Delivery service overlooked African American neighborhoods in major cities. Critics pointed out that the algorithm followed a long-standing pattern of avoiding economically redlined communities in order to optimize the "best" demographics to roll out the services. The investigation lead to a request from Boston Mayor Martin J. Walsh and Massachusetts Senator Ed Markey to Amazon to provide Prime Free Same-Day Delivery to Boston's Roxbury neighborhood, one of the traditional African American neighborhoods that was excluded.
Steady news of data breaches over the years has raised public awareness on technological mishaps. As a result, public curiosity has turned to how to best manage the power that algorithms can unleash. That curiosity has also turned into political action. New York City, for example, became the first city to pass legislation in which city government departments have to be transparent in using algorithms to deliver civil services, according to an Ars Technica post. A task force will examine whether city agencies appear to discriminate against people based on age, race, religion, gender, sexual orientation, or citizenship status.
Marketers should also be keeping abreast of how people are impacted by validation datasets used in classification models. Modeling techniques must fit the situation at hand. A random forest, for example, may work better than a neural network. Answering that question once encompassed purely statistical concerns, such as how many observations are needed. Now marketers must ask how representative is a technique for a real-world scenario.
Moreover, planning should explore how to make algorithm-influenced decisions understandable to the public or the audience impacted by the results. Those educational efforts can aid marketers efforts, too.
Making advanced analytics scientifically sound elicits technical steps. Making it ethically sound elicits social considerations. Marketers should express those social and technical concerns in a prime directive -- a governing principal much like the fictional Prime Directive made famous in Star Trek. On the show characters debate their decisions and technology exposed to cultures in various stages of development. In real life, marketers must highlight how norms and values are expressed in the systematic decisions from algorithms and data, using analytics to bridge those worlds of thought. Their work should also raise the question regarding how forward-thinking training data must be in order to minimize systematic social biases.
What's ultimately at stake for companies adopting non-biased machine learning is sales. Evidence shows that consumers link their values with their purchase decisions. For instance, in 2015 eMarketer reported that over half of consumers said they would stop buying from a company that they thought was unethical.
With systematic judgment infused into brand activity online and off, so much is at stake. Services based on algorithms must deliver the right opportunities and benefits to everyone. Getting to that fairness means recognizing when the data includes past social biases from human activity. Advancing a business now means asking if the data is really move society ahead.
About the Author(s)
You May Also Like
How to Amplify DevOps with DevSecOps
May 22, 2024Generative AI: Use Cases and Risks in 2024
May 29, 2024Smart Service Management
June 4, 2024