How to Cope with AI Disappointment
Have your first attempts at using artificial intelligence left you disillusioned? You’re not alone.
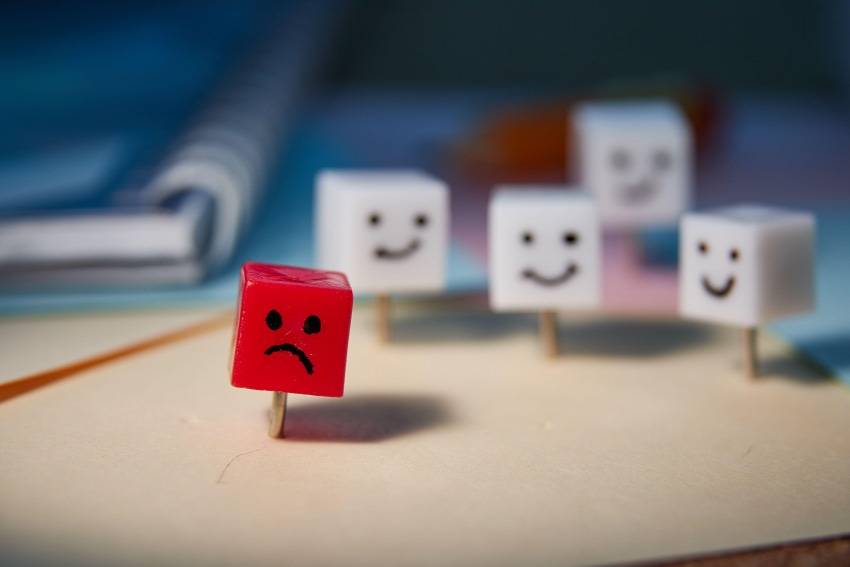
Artificial intelligence has captured the interest and imagination of millions of people and organizations worldwide. Now, after experimenting with AI, an untold number of early adopters are beginning to feel kind of let down as results fail to match once-optimistic expectations.
“The speed of GenAI adoption has left us in a Wild West of sorts where no one knows what the rules are, or should be, and unexpected behaviors and challenges burst onto the scene like a cowboy through saloon doors,” observes Joyce Tompsett, director of analyst relations at DevOps platform provider Digital.ai in an email interview. “AI projects often fail because they are untethered from the business, are attached to unrealistic or uninformed expectations, or lack the skilled resources needed to make the project successful.”
The Road to Regret
The goal of many early AI adopters is speeding up existing processes, Tompsett says. But then comes the inevitable speed bump when it turns out that the project wasn’t entirely thought through. What happens next? “Are you in clean-up mode, or are you focused on understanding what’s causing the problem?” she asks. It’s important to have the right human intelligence upfront to manage the AI and to build it into the process. “Enterprises want to implement AI to make programs faster, but must first understand the intended result, the true result, as well as the problems that haven’t been addressed.”
Two things can happen next. The overall project approach changes, and there’s a likelihood of increased problematic code. The additional throughput puts increased pressure on a part of the system that’s frequently already a bottleneck. “Without an updated process ... code churn and failure rates will increase, and inefficiency will reign,” Tompsett warns.
Too many AI projects launch with a focus on tools instead of working backward from a specific business challenge, says Ben Schreiner, Amazon Web Services’ SMB head of business innovation in an email interview. Start by focusing on a specific challenge, he advises. “Determine the size of that challenge. Identifying the benefit to the end customer and company is critical to achieving the right context and measurements of success,” Schreiner says.
Over time, technology acceleration and growth have led to increased innovation across multiple industries, a trend that remains true today, Schreiner says. “As AI gets out of the realm of research and into the real world a few experiments will fail,” he notes. “Still, others will succeed in empowering organizations and transforming operations, particularly for those with limited resources, like small and medium businesses.”
Regrouping
When facing a failed AI project, work to understand the underlying cause, Tompsett advises. In any event, don’t give up. “Iterate, iterate, iterate -- try something small and see if it works.”
Bruce Orcutt, CMO with intelligent automation company ABBYY, warns against using the popular large language models (LLMs) like generative AI in an initial project, which can prove costly and inefficient for many real-world business challenges. “To make AI adoption less daunting, managers should use purpose-built AI solutions that are intended for narrower, smaller-scale tasks,” he says via email. “Instead of throwing an alleged one-size-fits-all solution at an ambiguous set of business challenges, introduce purpose-built AI tools.”
Avoiding Future Disappointments
To prepare for unforeseen challenges, strong governance is necessary, as well as the ability to monitor processes and anticipate vulnerabilities. “One way to avoid disappointment is through continuous testing that involves constant trial-and-error in pre-production or internal projects before deployment,” Tompsett recommends. “Remember that the realm of the unknown surrounding AI is vast, so proactive risk management is crucial to protect IP and the bottom line.”
Enterprises, particularly SMBs, can avoid disappointment by ensuring they have the right infrastructure and support practices in place. “Businesses will need talent and technological capability to establish and execute a long-term vision that will create lasting value,” Schreiner says. Businesses must also create an IT infrastructure capable of supporting AI. “Since data is the lifeblood of AI, businesses should ensure their data is properly stored and accessible and that they have a data strategy that sets them up for success,” he explains. “By identifying a clear business need -- including reducing costs, increasing efficiency and productivity, generating novel insights from multiple data sources, or automating repetitive tasks -- businesses can best identify the right AI solutions.”
When all is said and done, despite occasional disappointments, AI isn’t something to fear, Schreiner concludes. “It opens many doors and, ultimately, will create opportunities we can’t even begin to imagine.”
About the Author(s)
You May Also Like