Is It Possible to Automate Trust?
First, businesses need a clear understanding of how data will be used, and who will be impacted by the decisions made using the data.
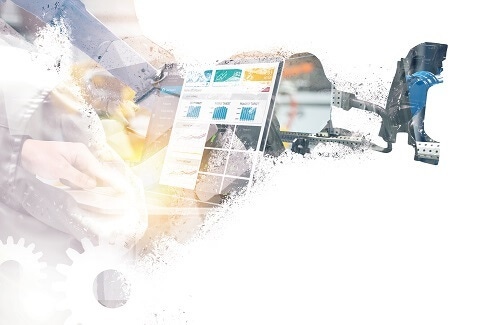
There is no shortage of new data and stories being shared on social media, broadcasted on television, or discussed among friends on Zoom or at socially distant gatherings. At a time when this already crowded environment is further inundated with news and messaging related to the current pandemic, it can be hard to decipher what is accurate.
Machines can potentially help us with this conundrum. Artificially intelligent minds can parse through millions of data points to find patterns and trends in a way that the average human cannot. With the right controls in place, artificial intelligence (AI) may be able to help us to automate trust -- and more quickly determine the accuracy and trustworthiness of the information.
How would automating trust work?
AI and other technologies can be used to automate trust and help consumers gauge the accuracy of information, a capability that is especially important during times like the current pandemic.
Technology can help consumers sift through a firestorm of information in a multitude of ways. Machine minds can cut down on the spread of false information thanks to their unique ability to parse through enormous sets of data at unprecedented rates. If we apply this to news stories, messages from business and other content produced around issues such as COVID-19, it can help identify falsities and stop the spread of misinformation in its tracks. AI and related technologies could also be utilized to calculate a trust factor: By quickly analyzing metadata related to a specific topic or source, AI could help consumers understand the trustworthiness of a piece of content by assigning it a “trust score” based on its origins, author history, and other factors.
To a certain extent, trust automation will always require some human oversight. What machines can do is speed up the calculation of a trust factor by supporting crowdsourcing of blind polls, using sentiment analysis of speech, or providing information about patterns in data sets. In some ways, automated trust already exists. When we go to a doctor, for example, we automatically assume that that doctor is going to keep our personal information safe.
That said, an extended use of automated trust to validate facts and cut down on the spread of false information will not happen unless people feel that the data used to inform is both unbiased and protected.
Challenges to automating trust
The biggest challenge to trust automation is that a big part of trust is perspective. Trust can be very subjective. For example, your own personal experiences may influence your viewpoint on any given topic without first considering any quantitative factors or data.
The lack of objectivity in trust aside, a more technical problem related to trust automation is of course, data bias. Biased data results in biased algorithms, which then become biased AI systems and other automated machines. This can hurt the communities the machines are supposed to serve. An example would be The Pittsburg model -- a system that was intended to help determine high-risk situations for foster children that ended up with implicit racial biases. If we do not confront the problem of data bias, good intentions could end up making the situation we were working to fix worse.
How can we safely automate trust?
To safely automate trust, businesses need a clear understanding of how data will be used, who will be impacted by the decisions made using the data and any potential for harm to create mitigation strategies.
To start, all developers on a team need to have a “kill switch” -- a virtual lever that can be pulled at any level of the organization if a bias problem is discovered. Giving teams this type of autonomy can seriously mitigate problems that might otherwise go unnoticed if decisions about bias are only being made at the highest level of an organization. To take it a step further, mitigating bias must start with onboarding, and anti-bias training should be required for any new hire, not just the developers.
Data security and stewardship is another essential element for automating trust safely. When it comes to data security and stewardship, rethinking governance is key. Governance in an organization is usually viewed as a project or a team that lives separately from the developers and analysts. In addition, businesses must create a system that incorporates governance in a way that establishes guard rails, allowing developers to do their best work. Embedding governance into the development process rather than existing as a separate entity or team can reduce chances of an “us versus them” mentality that often exists between security and compliance teams and developers. It also helps avoid a lot of scrambling and last-minute changes.
In a world of deep fakes and ample misinformation using AI, machine learning, and other emerging technologies can help customers understand what information to trust faster and help both brands and leaders cut through the noise to share accurate information.
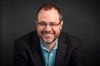
Sean Beard is a vice president at Pariveda Solutions, a consulting firm driven to create innovative, growth-oriented, and people-first solutions. Primarily, he works within Pariveda to evaluate and identify potential applications for emerging technology. His work involves a mix of consulting, research and development, and project-based tasks. He also self-identifies as a professional hobbyist -- he doesn’t just work with technology but considers it to be a lifestyle.
About the Author(s)
You May Also Like