My Algorithm is Better than Yours
New tools allow business users -- not just data scientists -- to leverage algorithms and venture into machine learning. But, handle with care because the choice of bad data means bad results.
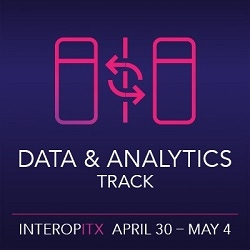
Machine learning is one of the hottest topics in tech today. It is a must-have organizational competency in the data-driven era of digital transformation. According to Deloitte's Technology, Media and Telecommunications Predictions 2018 Report, the number of machine learning implementations and pilot projects will double in 2018. IDC forecasts spending on artificial intelligence and machine learning will grow from $12B to $57.6B by 2021. The top industries adopting machine learning are retail, banking, manufacturing, and healthcare.
One of the key machine learning growth drivers is the improvements in augmented and automated user-friendly tools that can democratize the power of data science. Machine learning is no longer limited to doctorate level data scientists. Anyone can be empowered to rapidly extract value from data using a plethora of algorithms to make better decisions, act quickly and achieve better outcomes. However, buyer beware. It is also easier than ever to build bad models. How will you detect a good model from a bad model?
[Jen Underwood will be presenting a half-day workshop, Introduction to Machine Learning for Mere Mortals: Solving Common Business Problems with Data Science, during Interop ITX on Monday April 30 at the Mirage in Las Vegas. Click here to register using the code UNDERWOOD and save $300 on an All Access Pass or $200 off a Conference Pass.]
As machine learning algorithms are transparently weaved into business intelligence tools, automated decision-making processes, and the fabric of our day-to-day lives, it is becoming more important for everyone to understand the fundamentals. Machine learning is susceptible to a wide variety of bias types and a myriad of other issues if applied improperly. It also has amazing untapped potential when implemented correctly.
Basic machine learning concepts
For individuals with no prior knowledge of machine learning, McKinsey’s Executive Guide to Artificial Intelligence contains a high-level conceptual introduction to the basics of machine learning. The free online walk-through covers machine learning concepts, types and common use cases in simple, easy to understand terms.
For business and data analysts, most augmented and automated solutions leverage well-known, supervised, unsupervised and deep learning algorithm libraries behind the easy buttons. They merely simplify the machine learning life-cycle process in business user-friendly apps. The differences in algorithm performance can usually be evaluated and measured by precision, accuracy and other criteria. The input data format and quality provided to these new tools is usually the primary source of novice errors.
If an analyst or business user feeds an automated machine learning solution poor quality data, the predictive results will be poor. Thus, bad models are born. Think garbage in, garbage out. There still is an art to designing and providing data that accurately reflects a business process even if automated analytics can work through millions of variable combinations that would be unreasonable for a human to do. Only a human can understand and decipher nuances in business context.
Learning about machine learning
One way to get started with machine learning is to try open-source and free solutions, buy a couple books, take an online course, and explore your own data. There are many complimentary and low-cost resources available. If you are attending Interop ITX, I’ll be giving a fast-paced, half day workshop to introduce fundamental concepts and walk-through the entire machine learning lifecycle with optional hands-on exercises.
From selecting the right problem to solve to preventing algorithm bias, machine learning is still an art and a science. You’ll need to choose an appropriate problem that can be predicted with machine learning techniques. After identifying a suitable issue, then you’ll gather, understand, and prepare input data for algorithms to perform optimally. From there, you’ll begin experimenting with building machine learning models and testing if your model performs better than another model.
All in all, there are many potential variables in the machine learning modeling process. Rarely is only one machine learning model used in the real world. It is going to be incredibly difficult to decipher and sort through vendor claims of building better models. Most likely, you’ll still need to test with your own data to find out if that line of marketing FUD (fear-uncertainty-doubt) is fact or fiction.
About the Author(s)
You May Also Like