Predictive Analytics Troubleshooting: Identify Categorical Data Bias
Undetected data bias can render a launched predictive prototype model useless. don't let it happen to you. Here's a look at the biases possible and where marketers and agencies should start in their efforts to detect bias.
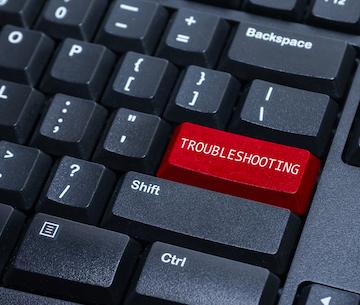
When you walk into a Las Vegas casino, knowing where to place your bets can be complicated. Another complicated process is knowing where your data bias is getting out of hand.
Not knowing about your data bias can have consequences with the advent of chatbots. Just ask Microsoft. Microsoft Tay, a chatbot, posted inflammatory and offensive tweets through its Twitter account, forcing Microsoft to shut down the algorithm only 16 hours after its launch. The decision tree for that bot contained a vulnerability that trolling Twitter users employed to corrupt Tay's responses into racist commentary.
But analysts are gaining more knowledge in ways to detect where bias occurs, particularly for clusters. Analysts are starting to learn how to break down the contributing elements in predictive models into segments to get a better view of bias factors.
I'll share a small tip from my experiences. The origins of the word "analysis" -- Greek for "break down" -- has been my rote explanation to business leaders for over 8 years. Back in my early years in analytics, I faced a huge effort to get everyone on the page for what web analytics was and the value it has to business.
Those initial discussions feel like a cakewalk now when I think about explaining the benefits of now widely available advanced analytics.
Analysts now must raise their capabilities as they dig into the data, identify errors, and put outliers into context against a model. The data hygiene needed for predictive analytics demands more than that of reporting dashboards. For example, very few models can handle empty fields. Predictive model errors scale quickly if the data used to train the model contains errors, so users should know how a model handles missing data before using it.
Categorical variables are another aspect that can impact data bias. These variables are binary in nature -- either something is in a given category or it is not. Thus categorical variables should draw from a data source with little variability.
But bias can appear even with something so binary as categories. Bias has significant consequences and is influenced increasingly come by real world experiences. A decision that presents opportunities based on stereotypes can be disastrous for a customer-facing business relying on chatbots. Chatbot designers should understand what questions may be influenced by culture, gender, and race in order to prevent catastrophes like the Microsoft Tay fiasco.
To get a handle on bias, first consider this modeling question -- how should you deal with outliers in a given dataset of expected categories? Is that outlier an error or an unknown data category that was not anticipated? Such questions help to frame context around a category.
That leads into a key tip. Know when data is influenced by conditions such as sales seasonality. That can help to highlight outliers or even spark debate among a business team if the seasonal data is appropriate for modeling.
It's best to incorporate responses to categorical concerns when you are applying data hygiene. Fortunately, the newest hygiene approaches are making best practices easier to establish. One example is tidy data, a concept first advocated by Hadley Wickham. Tidy data is a process to ensure that dataset columns, rows, and data appear in a consistent way prior to conducting deep analysis. In a preparation for data used for a cluster analysis, it can also assure that each cluster sees a sufficient data population once data has been arranged.
Simple models flush out categorical problems with greater highlighting when a model is run. A key quality on clustering is determining what will be the optimal number of clusters. That number is often not very clear from the data set itself. Thus selecting a trial with a simple model makes it easier to discern if the number of clusters should be adjusted. K-Nearest Neighbors, which assigns scores to data, is an example where a simple machine learning algorithm can be used.
A professional once wrote that the mystique of black box predictive modeling is giving way as more data -- and consequently, data bias techniques -- are being revealed. Analytics professionals can today decide the right technique to keep bias low and make the next Microsoft Tay a less likely bet.
About the Author
You May Also Like