The Dangers of Demonizing AI
As more individuals, governments and companies see artificial intelligence as evil, it becomes clear that we need metrics to ensure that AI is a good citizen.
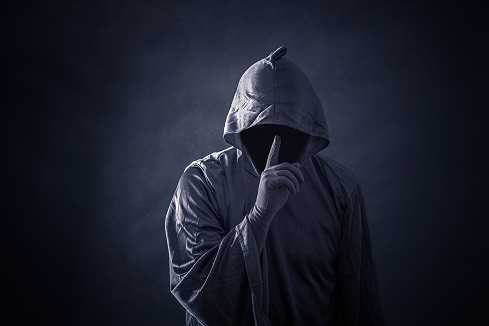
How do you benchmark the "evil" quotient in your AI app?
That may sound like a facetious question, but let’s ask ourselves what it means to apply such a word as “evil” to this or any other application. And, if “evil AI” is an outcome we should avoid, let’s examine how to measure it so that we can certify its absence from our delivered work product.
Obviously, this is purely a thought experiment on my part, but it came to mind in a serious context while I was perusing recent artificial intelligence industry news. Specifically, I noticed that MLPerf has recently announced the latest versions of its benchmarking suites for both AI inferencing and training. As I discussed here last year, MLPerf is a group of 40 AI platform vendors, encompassing hardware, software, and cloud services providers.
As a clear sign that standard benchmarks are achieving considerable uptake among AI vendors, some are starting to publish how well their platform technologies compare under these suites. For example, Google Cloud claims that its TPU Pods have broken records, under the latest MLPerf benchmark competition, for training of AI models for natural language processing and object detection. Though it’s only publishing benchmark numbers on speed -- in other words, shortening of the time needed to train specific AI models to achieve specific results -- it’s promising at some indefinite future point to document the boosts in scale and reductions in cost that its TPU Pod technology enables for these workloads.
There’s nothing intrinsically “evil” in any of this, but it’s more a benchmarking of AI runtime execution than of AI’s potential to run amok. Considering the degree of stigmatization that this technology is facing in society right now, it would be useful to measure the likelihood that any specific AI initiative might encroach on privacy, inflict socioeconomic biases on disadvantaged groups, and engage in other unsavory behaviors that society wishes to clamp down on.
These “evil AI” metrics would apply more to the entire AI DevOps pipeline than to any specific deliverable application. Benchmarking the “evil” quotient in AI should come down to a matter of scoring the associated DevOps processes along the following lines:
Data sensitivity: Has the AI initiative incorporated a full range of regulatory-compliant controls on access, use, and modeling of personally identifiable information in AI applications?
Model pervertability: Have AI developers considered the downstream risks of relying on specific AI algorithms or models -- such as facial recognition -- whose intended benign use (such as authenticating user logins) could also be vulnerable to abuse in “dual-use” scenarios (such as targeting specific demographics to their disadvantage)?
Algorithmic accountability: Have AI DevOps processes been instrumented with an immutable audit log to ensure visibility into every data element, model variable, development task, and operational process that was used to build, train, deploy, and administer ethically aligned apps? And have developers instituted procedures to ensure explainability in plain language of every AI DevOps task, intermediate work product, and deliverable apps in terms of its relevance to the relevant ethical constraints or objectives?
Quality-assurance checkpointing: Are there quality-control checkpoints in the AI DevOps process in which further reviews and vetting are done to verify that there remain no hidden vulnerabilities -- such as biased second-order feature correlations -- that might undermine the ethical objectives being sought?
Developer empathy: How thoroughly have AI developers considered ethics-relevant feedback from subject matter experts, users, and stakeholders into the collaboration, testing, and evaluation processes surrounding iterative development of AI applications?
To the extent that these sorts of benchmarks are routinely published, the AI community would go a long way toward reducing the amount of hysteria surrounding this technology’s potentially adverse impacts in society. Failing to benchmark the amount of “evil” that may creep in through AI’s DevOps processes could exacerbate the following trends:
Regulatory overreach: AI often comes into public policy discussions as a necessary evil. Approaching the topic in this manner tends to increase the likelihood that governments will institute heavy-handed regulations and thereby squelch a lot of otherwise promising “dual-use” AI initiatives. Having a clear checklist or scorecard of unsavory AI practices may be just what regulators need in order to know what to recommend or proscribe. Absent such a benchmarking framework, taxpayers might have to foot the bill for massive amounts of bureaucratic overkill when alternative approaches, such as industry certification programs, may be the most efficient AI-risk-mitigation regime from a societal standpoint.
Corporate hypocrisy: Many business executives have instituted “AI ethics” boards that issue high-level guidance to developers and other business functions. It’s not uncommon for AI developers to largely ignore such guidance, especially if AI is the secret sauce for the company to show bottom-line results from marketing, customer service, sales, and other digital business processes. This state of affairs may foster cynicism about the sincerity of an enterprise’s commitment to mitigating AI downsides. Having AI-ethics-optimization benchmarks may be just what’s needed for enterprises to institute effective ethics guardrails in their AI DevOps practices.
Talent discouragement: Some talented developers may be reluctant to engage in AI projects if they consider these a potential slippery slope to a Pandora’s box of societal evils. If a culture of AI dissidence takes hold in the enterprise, it may weaken your company’s ability to sustain a center of excellence and explore innovative uses of the technology. Having an AI practices scorecard aligned with widely accepted “corporate citizenship” programs may help assuage such concerns and thereby encourage a new breed of developers to contribute their best work without feeling that they’re serving diabolical ends.
The dangers from demonizing AI are as real as those from exploiting the technology for evil ends. Without “good AI” benchmarks such as those I’ve proposed, your enterprise may not be able to achieve maximum value from this disruptive set of tools, platforms, and methodologies.
To the extent that unfounded suspicions prevent society as a whole from harnessing AI’s promise, we will all be poorer.
[For more on AI check out these recent articles.]
Human Capital Management Technology May Be 'Demo Candy'
About the Author(s)
You May Also Like