Traits That Make A Great Data Scientist
Not everyone has the chops to cut it as a data scientist. Here are the skills that matter most, says tech consulting firm Booz Allen Hamilton.
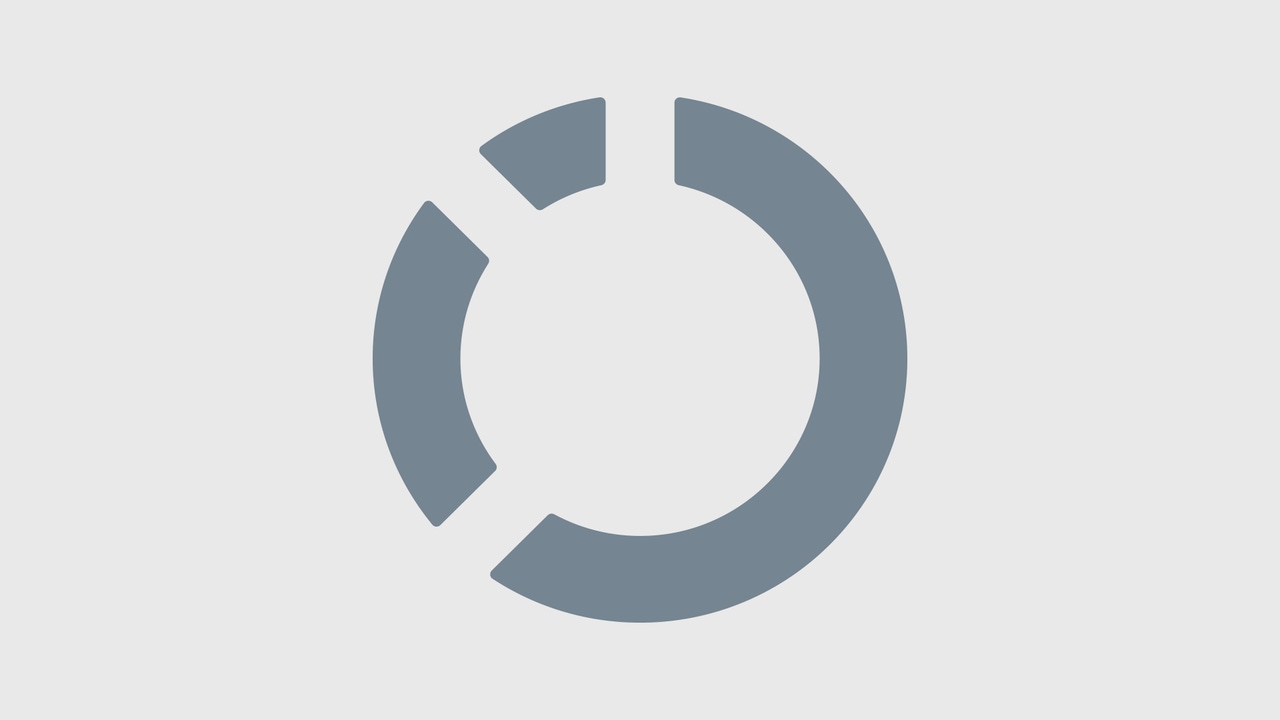
10 Jobs Destined For Robots
10 Jobs Destined for Robots (Click image for larger view.)
Frequent readers of InformationWeek's big data coverage are well aware that a successful data science program requires a team of trained experts, each with a unique skill set, rather than one unicorn employee capable of doing it all.
But knowing that doesn't necessarily simplify the task of hiring qualified data scientists. Booz Allen Hamilton's "The Field Guide to Data Science" is a 110-page primer that goes into great detail on how to build a data science team. We've summarized some salient details below.
When building your team, it's important to focus on these key qualities:
Curiosity: Required to "peel apart" problems and study relationships between data, including those that may at first glance seem unrelated.
Creativity: For devising and attempting new problem-solving approaches and potential solutions that haven't been tried before.
Focus: Essential for designing and testing techniques over lengthy periods (days or weeks). A tenacious attitude is important for learning from failure and trying until you get it right.
Attention to detail: Important for maintaining rigor and for avoiding an over-reliance on intuition when analyzing data.
[Give your interviewer the answers they deserve. See 16 Stupid Tech Job Interview Questions: Show Your Snark.]
Of course, proficiency in key technical disciplines is required, too, specifically computer science, domain expertise, and mathematics.
A computer science background is essential for data processing and manipulation. Advanced math skills, including a solid background in calculus, geometry, linear algebra, and statistics, are required for understanding the basis for algorithms and other data science tools, the report said. And domain expertise helps the data scientist understand the problem at hand and how to measure it.
Where do you find data scientists? Your first impulse may be to look outside of your organization for qualified candidates, but the Field Guide recommends first looking in-house for people "who have a high aptitude" for data science.
Potential team members will likely have advanced degrees in the three technical areas described above, but you shouldn't immediately dismiss candidates who don't.
"Don't discount anyone -- you will find data scientists in the strangest places with the oddest combinations of backgrounds," the report states.
Leadership skills are important, particularly for the first members chosen for your team.
One common weakness of data science teams is an inability or unwillingness to imagine new and different approaches to problems. You'll want to foster an environment of openness, one that encourages "trust and communication across all levels, instead of deference to authority." Managers should encourage data science team members to speak up and ask questions frequently.
In addition to building the team, you'll need to choose an operating model. The Field GuideĀ suggests three options:
A centralized team that works under a chief data scientist and serves the analytical needs of the entire organization
Smaller data science teams deployed to specific business groups for short- or long-term assignments
Diffused teams embedded over the long term within each business group
Political, not technical, problems are often the biggest challenges facing a data science unit, particularly if management is ambivalent about the team's mission.
To prove its value, a team "needs to initially focus on the hardest problems within an organization that have the highest return for key stakeholders." Doing so can change (ideally for the better) how the organization approaches future challenges.
A data science team also needs support from management to lessen in-house "fears and doubts" about its mission. Leaders must be strong advocates to ensure "widespread buy-in" of the team's objectives, the report says.
Jeff Bertolucci is a technology journalist in Los Angeles who writes mostly for Kiplinger's Personal Finance, the Saturday Evening Post, and InformationWeek.
You can use distributed databases without putting your company's crown jewels at risk. Here's how. Also in the Data Scatter issue of InformationWeek: A wild-card team member with a different skillset can help provide an outside perspective that might turn big data into business innovation. (Free registration required.)
About the Author(s)
You May Also Like