Trump's Election: Poll Failures Hold Data Lessons For IT
Are your data analytics predictions models suffering from the same problems as the models that predicted Hillary Clinton would easily win the US Presidential election? Here are a few things to consider.
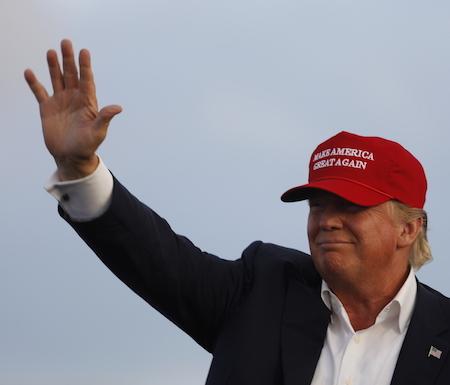
8 Ways To Ensure Data Quality
8 Ways To Ensure Data Quality (Click image for larger view and slideshow.)
How good is the data we rely on in our lives and businesses? After hundreds of polls were conducted and analyzed to predict who would win the US presidential election, the data scientists, statisticians, and media outlets that analyzed those polls failed to predict that Donald Trump would win against Hillary Clinton on Election Day. It was a shocker to many.
That brings up an important question for IT organizations that are investing in data and tools to analyze it. How good is that data? How good are those insights? Can we trust them? How can we make our predictive models more accurate? What lessons can we learn from the polling data issues that can be applied to our own organizations' data efforts?
[What did FiveThirtyEight's Nate Silver say about the presidential election a year ago? Read Nate Silver Predicts 2016 Presidential Race at Salesforce World Tour.]
The first thing to do is ensure the quality of the data.
"Polls are just like any other analytical model," said Bennett Borden, chief data scientist at the law firm Drinker Biddle & Reath, in an interview with InformationWeek. You have to ask, "Where are you getting your data from? Clearly there was a whole swath of the population that we did not reach in this polling data."
Borden followed election polling on the FiveThirtyEight media site headed up by Nate Silver, who won fame for correctly predicting the 2008 election. The trained statistician subsequently released a bestselling book, The Signal and The Noise: Why So Many Predictions Fail but Some Don't.
Silver's site was the most pessimistic about a Clinton win, but it still had her as the favorite in the election based on internally created models that incorporated voter polls. Clinton's lead was small enough to be a polling error.
Borden said that the other problem that can happen with polling data is that people don't always provide answers that reflect their true opinions. He said that that was a concern he'd heard expressed recently in a presentation by Chicago Mayor Rahm Emmanuel who had said his biggest fear about the polling data was that there were people who were afraid to admit in the polls that they were going to vote for Trump. That's something that has been cited in other coverage, too.
"It's like the Nielsen ratings. When people would write down what shows they watched, they always ended up watching documentaries on PBS, when in reality they were watching the Simpsons," Borden said.
That kind of embellished reality from respondents makes collecting accurate data more difficult when doing polls.
"It shows when you are gathering data to build your models on, you have to make sure the data is accurate," Borden said. "We think analytics are some kind of magic. Overreliance on analytics without understanding their limitations is where we see some of the issues coming in."
A recent survey by consulting firm KPMG recently found that while organizations are investing in data and analytics, they don't always place a high degree of trust in the results.
The firm's UK director of global data and analytics, Nadia Zahawi, told InformationWeek in an interview recently that these analytics efforts often reside in a "black box." Data goes in, insights come out, and stakeholders never see what happens inside.
KPMG's report said other drivers, too, contribute to mistrust. For instance, decision-makers may be suspicious of the motives or abilities of internal or external expertise. Or they may subconsciously feel that their successful past decisions justify continued use of old sources of data and insight, leading to what KPMG says is a form of cognitive bias.
Drinker Biddle & Reath's Borden notes that algorithms that replace these insights can sometimes end up encoding the cultural biases and confirmation bias that organizations are trying to fix.
For instance, if machine-learning training data looks at all the successful managers in an organization's history to reveal insights about a profile for those managers, the resulting algorithm to find those managers will likely identify white men as the best candidates, because they are the people who enjoyed management success in the past.
It's a danger for future development efforts, and society has not yet really begun to address the governance and regulatory challenges posed by this danger, Borden said.
About the Author(s)
You May Also Like