What Can a CIO Do About AI Bias?
In a world filled with bias, can AI algorithms ever truly be unbiased, and how can IT leaders address technologies' blind spots? Transparency may be key.
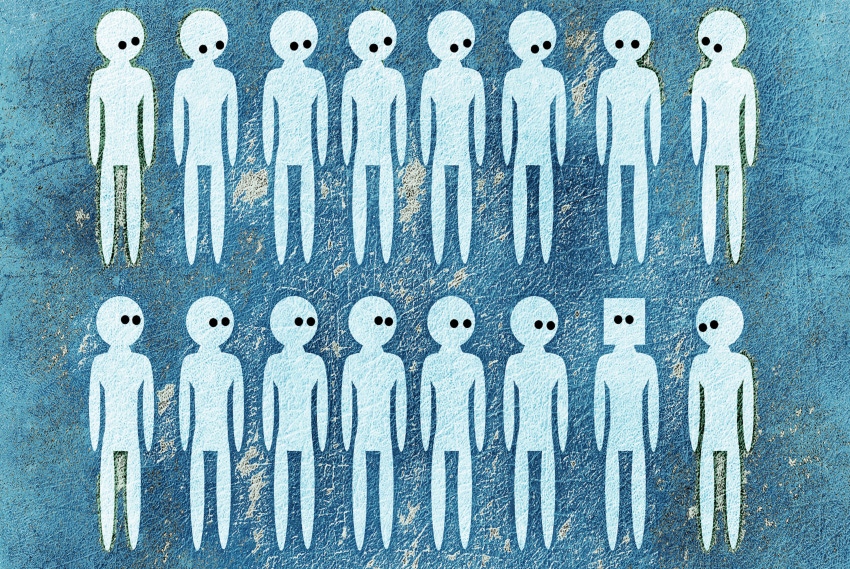
Although AI algorithms provide the capabilities to predict patterns, in many industries they also bring unfortunate downsides -- such as exacerbating the impacts of existing bias. Some AI algorithms slant data in favor of one group or another, and that can bring discrimination when it comes to job applications and mortgage approvals, as well as eligibility for healthcare treatments and university admissions.
The examples are piling up. How can organizations recognize when the technology they’re using is discriminatory, and what do they do about it?
Bias In, Bias Out
Last year, finance and human resources application cloud provider Workday was sued in a class action complaint for allegedly building artificial intelligence (AI) job-screening tools that caused bias against Black job applicants in their 40s. The US District Court for the Northern District of California dismissed the case on Jan. 19, 2024, for insufficient evidence. But plaintiff Derek L. Mobley filed an amended complaint on Feb. 20 saying Workday’s algorithmic-based application screening tools were biased against people based on race, age, and disability. Mobley had been rejected for more than 100 jobs he applied for while using Workday’s software, according to reporting by Reuters.
In a statement, a Workday spokesperson told InformationWeek: “We believe this lawsuit is without merit and deny the allegations and assertions made in the amended complaint. We remain committed to responsible AI.”
Meanwhile, an investigation by nonprofit news organization The Markup revealed that 80% of Blacks, 40% of Latinos, and 70% of Native Americans were likely to be turned away for home loans due to AI bias.
In machine learning, there is a trend of “garbage in, garbage out,” notes Daniel S. Schiff, assistant professor in the Department of Political Science and co-director of the Governance and Responsible AI Lab (GRAIL) at Purdue University. He explains that if data is coming from a biased world, then the data will replicate that bias, Schiff says. This concept can apply to employment patterns as well as loan approvals, according to Schiff.
“If you've only given loans to people X or only hired people like people Y, your model might just sort of reproduce that, because it's trained to think those are the matches,” Schiff says.
Algorithms turn data into models, and the models bring about predictions, which could be biased, Schiff explains.
“If the predictions immediately have apparent biases, it could be the data or it could be the algorithm,” Schiff says. “There are ways we can sort of constrain the model to act in better ways.”
Experts such as Fred Morstatter, research assistant professor of computer science at the University of Southern California, believe that all AI is biased. Gaurav Agarwal, CEO and founder of AI testing platform RagaAI, also sees AI bias as ubiquitous, including large language models (LLMs), generative AI, and computer vision, which video surveillance cameras and facial recognition technology incorporate.
“Anywhere we are seeing AI deployed, we are seeing some form of bias,” Agarwal tells InformationWeek. “I would say it is extremely prevalent.”
How to Find and Fix AI Bias
USC has been working to measure how to achieve statistical equity while using AI and identify biases in LLMs, Morstatter says. To measure bias, USC uses prompting and glass-box methods, which are completely transparent to users and easily explainable compared with black-box AI.
The university used a group of algorithms called quality-diversity (QD) algorithms to fill gaps in training data and create more diverse data sets. It built a diverse dataset of about 50,000 images in 17 hours. By diversifying the data set, the research group produced improved accuracy on faces with darker skin tones, lead author Allen Chang, a USC senior, said in a blog post.
Tools such as IBM’s AI Fairness 360 (AIF360) can tell IT leaders to what degree their AI models are biased toward one group of people versus another. RagaAI’s tool, called RagaAI DNA, identifies biases in AI models by training on a multimodal data set. Organizations can custom-train RagaAI DNA to test product descriptions and customer reviews in retail, location data in geospatial applications, and medical records and images in healthcare.
In addition, the Google What-If Tool analyzes the behavior of trained machine learning models.
What to Do When You Realize AI Is Biased
When IT leaders discover that they are using biased AI, they must retrain or reteach the algorithms, explains Dr. David Ebert, director of the Data Institute for Societal Challenges at the University of Oklahoma. In addition, they must reevaluate the decisions that were based on AI algorithms.
Companies should systematically diagnose the origin of the issue, including where AI is building and outputting data, Agarwal says.
In addition, IT leaders should use training data that is relevant for multiple cultures, Agarwal suggests. In one example, ChatGPT showed bias toward certain cultures when it gave incorrect tipping advice for Spain, which trends lower than tipping rates in the United States.
“The bias came because the humans that used to train the data were primarily looking for data from a specific culture,” Agarwal says.
He explains how algorithms in cars’ video cameras may not be able to detect all weather conditions because at times they only work well in good lighting during the day but not well at dawn or dusk.
“It was able to detect cars and pedestrians during a good day but not as well during low lighting, evenings, for example,” Agarwal says. “The AI in this case works very similar to what humans were able to see.”
Training AI can help overcome this glitch, according to Agarwal.
“It was a data imbalance issue and a model training issue,” Agarwal says. The automaker used RagaAI’s tool to diagnose and fix this bias.
Auditing, Transparency, and ‘Sustainable Sourcing’
Transparent labeling can expose AI bias. Just as food products in a grocery store have food labels with ingredients and nutrition information, AI products should also have similar transparency and explanations, Ebert says. This transparent labeling could help expose AI bias.
“Similarly, standards for training and explanations can be created so that a user knows when they are using an AI that is trustworthy for the data they are examining,” Ebert says.
To avoid adopting an AI tool that turns out to be biased, Agarwal recommends “sustainable sourcing,” in which tech leaders must ensure that they are sourcing legitimate tools, according to Agarwal.
“If I'm the CIO or CTO who is sourcing this AI, I want to make sure that my vendor has all the right checks and balances, all the right processes, and all the right tools in place to develop their own AI, which is not biased,” Agarwal says. “I think that's very important.”
IT leaders must do background checks and research the AI tools they deploy and use bias-assessment frameworks, Agarwal says.
Once companies deploy AI, they need to perform continuous auditing and monitoring to ensure that the AI does not contain bias. Companies should also make avoiding AI bias as important to an organization as a diversity, equity, and inclusion (DEI) charter, Agarwal advises.
To avoid AI bias, ensure that the data being entered into an AI process is consistent with the characteristics of training data, according to Ebert.
“For instance, if a machine learning algorithm to predict sales of air conditioners was trained based on sales in Chicago and you are applying to your data for Las Vegas, you may get biased and incorrect predictions,” Ebert says.
Companies need to ensure that data is representative of diverse populations, Schiff says.
“If you have a data set and it's trained on only white male faces, it's probably not going to be as good at imaging medical diagnosis as if it had more populations,” Schiff explains. “So you might try to gather more representative data or make sure that it's relevant to the population you're serving.”
Meanwhile, IT leaders can check the AI Incident Database to see if a tool they plan to use has been reported to have AI bias.
As part of an auditing process, business leaders can speak with multiple stakeholders and subject matter experts to discuss the coding behind algorithms to test for well-being, human rights, or algorithmic impact, Schiff says.
“Essentially there's a deep auditing and thinking process that you need in addition to some of the quick technical strategies that can catch some key things,” Schiff says.
In addition, companies should have a risk management or governance structure in place to ensure that the design and implementation of AI models is responsible, Schiff advises.
For guidance, companies can consult the NIST Risk Management Framework (RMF), Schiff says. Schiff calls RMF the “most robust US effort” to combat AI bias. Companies can also consider hiring ethicists or policy experts to regulate AI biases.
The IEEE has an Algorithmic Bias Working Group to guard against bias. NIST recommends that industry sectors create individual “profiles” for LLMs. Government agencies for transportation and healthcare have their own guidance on AI, and they will move from guidance toward more formal rules, Schiff predicts.
When evaluating AI tools, maintain some skepticism, Schiff advises.
“If anyone says, ‘our products are bias-free,’ that's a red flag,” Schiff says. “It's not how things work.”
When companies can verify that an organization such as IEEE has audited their AI tools or they comply with the European Union’s AI Act, then they can be considered fair or legitimate, Schiff suggests.
Read more about:
Diversity, Equity, and Inclusion (DEI)About the Author(s)
You May Also Like