What Enterprises Have to Learn About Emerging Tech, Again
Enterprises often make the same mistakes when adopting technology, generation after generation. A PwC tech leader outlines seven considerations to keep in mind.
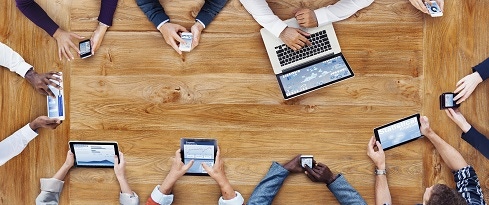
New technology is always emerging. Yet, enterprises don’t always learn from past mistakes. As new generations of technology replace their predecessors and completely new technologies hit the scene, enterprises must adapt, though old habits die hard. Heading into 2019, following are a few things business and IT leaders should keep in mind.
#1: Business as usual isn’t static. Organizations continue to become more digital, but not all of them are continually reassessing whether their operating and risk management models are still relevant. Customer preferences change, business practices evolve, and, in some cases, the nature of emerging technologies shifts.
“The pace of change is accelerating which challenges our clients,” said Scott Likens, new services and emerging technology leader at PwC. “Before, you could have an intelligent plan around the next big technology. In the 1990’s, ERP, Y2K and dot coms were happening. While [all of that involved] emerging tech, it was very plannable. Now we’re in a world where it’s unplannable.”
As a result, businesses need to embrace Agile, iterative ways of working that expedite trial and error cycles and to enable more to be tested in parallel.
#2: Silver bullets are only silver-plated. The latest, greatest technology still tends to be viewed as a silver bullet. Regardless of what it is – computers, networks, enterprise applications, the Internet, mobile, cloud, AI, blockchain – the shiniest new technology isn’t the thing that’s going to make a company invincible.
“The reality is that AI and blockchain are high-risk because they’re changing fast and the technologies are unknown,” said Likens. “With AI specifically, there’s a lot of risk in the opaqueness of some of it, so being responsible about this technology is something we’re passionate about. [It’s important to put] guardrails around responsible AI or responsible innovation.”
#3: Rigid, long-term plans are for dinosaurs. As the pace of technology innovation, adoption and disruption continue to accelerate, it becomes harder to anticipate what any business will look like over the long-term, which used to mean five years in the U.S. and 50 years in Japan, for example.
“In the past, you kind of knew what all of the technology trends were because we were looking at core systems, modernization, and data warehouses. All of that has kind of blown up because of the cloud, access to data, emerging tech, and embedding intelligence into these living breathing roadmaps,” said Likens. “Trying to guess a five-year technology roadmap is kind of comical now. You want to have a vision with a high-fidelity six months and a lower fidelity 18 months.”
It’s not that business planning is obsolete. It’s that the plan-execute-evaluate process no longer has a multi-year cycle. Now the trend is fast iteration.
“No matter the industry, [if you have] a five-year plan, year one is great, year two starts to erode, year three everyone asks, ‘What are we doing?' ” said Likens. While a vision and plan are still important, they need to be balanced with an organizational ability to pivot with the times.
The upside of some of today’s emerging technologies like AI, mixed reality and blockchain is that their application is narrower than core enterprise systems. Therefore, smaller-scale experimentation can occur in shorter time frames.
#4: Annual budgets and tech adoption align even less now. Thanks to widely-accepted accounting standards such as Generally Accepted Accounting Principles (GAAP) and International Financial Reporting Standards (IFRS), companies still operate based on annual budgets, even though technology adoption cycles have been diverging from that model for some time. The fact that businesses are becoming ever more digital is not reflected in those accounting standards, so organizations need to find a way to bridge the gap between the two.
“The annual cycle is something we have to break from a funding perspective,” said Likens. “When you get into emerging tech and you start talking about two-week sprints and continuous innovation, you never say annual budget and you have to break down that barrier.”
Instead of releasing an annual budget all at once, organizations can release pieces of that budget and continue to invest based on outcomes. The annual budget still exists for accounting purposes, but the investment model becomes iterative to better align with technology investments.
#5: Transformation isn’t just technological. Digital transformation and all the emerging technologies that feed into it aren’t just something for the IT department to ponder. Technology is integral to the success of the business, so it needs to be approached that way. That means, framing emerging technology in terms of its potential opportunities and risks to the business rather than framing it as a technology problem for the IT department to solve.
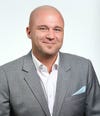
Scott Likens, PwC
“That’s something we missed in technology transformations of the past. We’ve just focused on this core that we’re changing, not the change across the organization around people,” said Likens. Business operating models need to evolve with the times, which means greater user empowerment across the organization and democratized forms of innovation.
“In 2000 emerging technology was on the side. It was let‘s build our ecommerce capability or let‘s build our digital business,” said Likens. “Now every business is digital. The question is how to continually infuse that, so the operating model has to change.”
#6: ROI may be harder to quantify. GAAP and IFRS rely on tried and true methods of valuing physical assets; however, the same is not true for intangible assets. Although organizations are starting to see value in the IP of an algorithm, the algorithm itself may be less valuable than thought. To deliver value, the algorithm has to be trained in a manner that is specific to its use, and the data fed into the algorithm will determine whether the algorithm is more or less accurate.
“It‘s hard to draw the line of where the value is in the data versus the algorithm in the outcome,” said Likens. “The value is hard to draw a box around, because there’s value in every piece of the puzzle. There’s inherent value in the outcome and that’s quantifiable. What’s not quantifiable is what it took to get to that outcome.”
Most people don’t yet realize that for machine learning to be effective, people still need to annotate the data, which is a very manual effort. Despite claims that AI and raw data are all that’s needed to achieve magical outcomes, good old data engineering is still required.
“Using and annotating and getting the data fed into the algorithms is the hard part. You have to understand the domain of that data or else the mathematics don’t matter,” said Likens. [Previously,]
we made a run at AI with purely math which was cool but it didn’t affect the business. Now we’re in the era of mixing the business with the math and that’s really important but very hard from a skill perspective.”
#7: Emerging technologies may be more than “just another technology.” AI is often considered just another technology since new technologies are always coming down the pike. However, self-learning systems differ from deterministically programmed systems. The failure to recognize the shift from deterministic systems to probabilistic systems is an inherent flaw in today’s risk models.
Organizations should also think more critically about blockchain.
“[Blockchain] says don’t land data, spread data. [When you consider] regulation or privacy, it’s an opposite architecture [because] blockchain says everyone should have the data, so how do we leverage both at once?” said Likens. “When you mix AI and blockchain, there’s a lot of upside there but not a lot of precedents around that tell us how that’s going to happen.”
In short, new technology and new versions of technology are constantly emerging, but the approach to technology adoption, operating models, and risk models need to evolve with the times. Old habits die hard, but with the pace of change constantly accelerating and the nature of some technologies bringing with them unintended consequences, critical thinking isn’t optional, it’s essential.
[For more about today's emerging technologies and concepts, check out these recent articles.]
Top 10 Tech Predictions for 2019
AI in Production: A Roadmap for Success
Cloud Trends: Look Behind the Numbers
6 Top Emerging Technology Jobs for 2019
Predictions for Artificial Intelligence in 2019
About the Author
You May Also Like