Beware the Pitfalls of Applying AI to Big Data
Decision makers should consider whether conclusions are influenced by shortcomings in the observable data and whether the algorithms properly address biases.
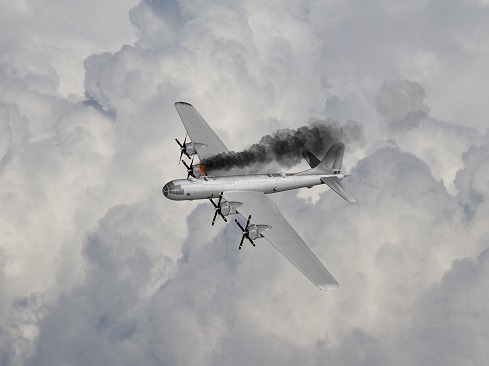
Artificial intelligence is increasingly being applied to large volumes of real-world, observational data to answer questions on everything from understanding human behavior to optimizing business processes. The unfortunate reality, however, is that most observational data is “dirty,” and whether AI ultimately realizes its enormous potential will depend on the care taken to understand and address data biases.
The importance of data quality can be illustrated through two contrasting examples. First, Billy Beane’s Moneyball strategy in Major League Baseball demonstrated how players can be valued using the insights derived from statistical analysis of rich and accurate data on in-play performance.
An opposing example dates to World War II, when Abraham Wald, a statistician, was asked to examine data on B-29 bombers riddled with bullet holes and prescribe where to apply additional armor. Since fuselages took much of the fire, reinforcing them seemed the obvious prescription. Wald, however, recognized that the observable data was “dirty,” because it had been compiled from surviving bombers. Bombers that were hit in places that were fatal, such as the engine, did not make it back and had been omitted from the data. Accordingly, Wald’s recommendation to apply the additional armor to sections that had the fewest bullet holes -- especially the engine -- ultimately saved countless lives. This is an example of selection (specifically survivorship) bias, and why it is important to account for it to avoid erroneous conclusions.
A more recent example of selection bias is Amazon’s use of an algorithm to vet resumes based on data from past hiring decisions. Amazon recognized that the algorithm gave undue preference to men, but even after gender was excluded from the selection criteria, the algorithm continued to penalize female candidates by circumventing the restriction by finding gender proxies (e.g., “sorority”). Ultimately, Amazon abandoned the algorithm.
A further example is Microsoft’s Tay, an AI-based Twitter chatbot launched in 2016, designed to interact with and learn from Twitter accounts to engage in “casual and playful conversation.” In less than 24 hours, however, Tay began tweeting offensive material, highlighting that many of the “conversations” on Twitter are not representative of “casual and playful.”
Another type of bias is confounding bias, which occurs when an apparent association between two variables is caused by the presence of an additional confounding variable. For example, a person’s income and education are likely to be positively correlated, but both may be affected by confounding variables -- intelligence or work ethic -- which may be harder to capture. Failing to address the confounding effects may result in erroneous associations that can lead to improper conclusions.
For example, confounding bias afflicts many health studies that report surprising associations between positive health scores and consumption of potentially unhealthy foods. One study found that people who drink alcohol had fewer heart attacks than those who abstained. However, poor health may cause people to abstain, and failing to control for that effect yields spurious associations and misleading comparisons between groups.
Recommendations
First, examine the data through the lens of causality. Focusing on potential causal relationships between variables assists in assessing data quality, recognizing missing variables, and identifying pitfalls, such as selection and confounding biases.
Second, apply appropriate statistical techniques. Selection bias has been studied extensively in econometrics since James Heckman’s pioneering work for which he won the Nobel Prize in Economics. Publications related to machine learning (ML), a subset of AI techniques, have sought to address selection bias through reweighting of the data. A review of ML-related patents addressing selection bias also focuses on reweighting. For example, a patent assigned to Amazon describes a negative weighting scheme to offset selection bias that arises when recommendations are presented to users. A patent assigned to IBM describes a weighting procedure that addresses selection bias when using movie reviews to predict reviews of non-movie products.
In dealing with confounding bias, economists often rely on using instrumental variables in simultaneous-equations models. An example of this technique being applied comes from a study examining return on investment (ROI) from online advertising spending. Under conventional analysis, the ROI was a striking 1600%. Recognizing, however, that searching reflected an intention to buy, the study controlled for this confounding effect, and after correction, found no causal relationship between online ads and purchases, suggesting that most consumers would have purchased without the ads.
Third, place less emphasis on prediction accuracy. In many contexts, economists regard prediction accuracy as secondary to understanding causal effects. As an example, a simultaneous-equations model to understand causality will often have lower predictive power than simpler predictive models.
AI is yielding insights in an ever-growing number of areas, but decision makers must be aware of the potential pitfalls with observational data. Decision makers should consider whether conclusions are influenced by shortcomings in the observable data and whether the algorithms properly address biases.
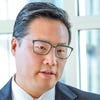
William Choi is a Managing Director in the economics consulting business at AlixPartners, based in San Francisco. He has published and testified as an expert witness on statistical methods. He also has advised companies on how to better leverage their datasets.
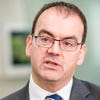
Mat Hughes is a Managing Director in the economics consulting business at AlixPartners, based in London. He has written on the application of Big data in insurance markets, the assessment of price signaling under competition law and the assessment of cartel damages.
About the Author(s)
You May Also Like