Does AI Competence Matter?
AI and machine learning are becoming more commonplace, but the people using such systems may not be qualified to operate them.
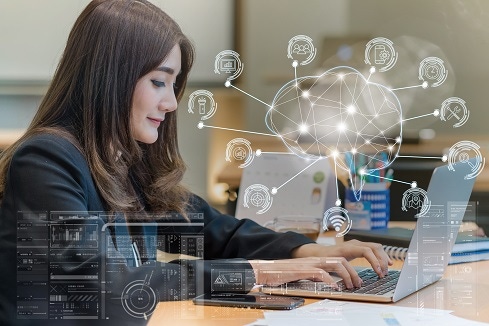
AI is being built into more systems and software as organizations attempt to compete in the algorithmic age. With the level of machine intelligence reaching new heights, the number of experts is not growing proportionally. To compensate, AI libraries, APIs, systems and software are becoming easier to use so more people can take advantage of them. However, ease of use does not necessarily diminish risks.
At present, there's no minimum competence level one must have to operate an AI system, except perhaps data scientists with graduate degrees in math, statistics or computer science who use the most sophisticated tools. While there are AI-related nano degrees and certificates for technologists and business leaders, there's no central licensing or certification entity that everyone trusts, at least yet.
Time to market isn't everything
Earlier this year, Gartner reported that 37% of the 3,000 CIOs surveyed were either implementing AI or would be doing so soon. A newer study by Dun & Bradstreet showed that 83% of finance teams at leading finance and credit lending companies in the U.K. and U.S. are automating at least one part of their processes.
Granted, not all AI systems are alike. Some of them are relatively "dumb," because they use pre-determined inputs and outputs. However, even simple systems need to be monitored and updated. For example, a company building a customer service chatbot will typically want to expand the list of questions the bot is capable of answering.
More sophisticated systems use machine learning or deep learning to unearth patterns or signals in data. Those systems also require ongoing attention, albeit on more levels. For example, the data used for machine learning training tends not to be static and data quality is important. As new data comes in, the model must be tuned to ensure its ongoing accuracy. Or, to meet a different business goal, an organization might use different data, algorithms, and models.
"Where we've had success is where we've been able to bring machine learning or AI to [clients] and basically ingest the data that relates to their accounts receivables with the data we have and give them quick results they can act on," said Andrew Hausman, general manager, Financial Solutions at Dun & Bradstreet. "We go back to clients several times a year and fine-tune the results based on what they want to see: For example, if somebody says we want to grow more sales, we want to extend more credit to clients or we want to be more risk averse because a recession is coming."
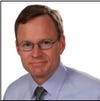
Andrew Hausman
AI experts understand what can go wrong with AI and why, but they're in the minority. The reason broader AI competence is important is that machine learning and AI can impact individuals, groups and societies in profound ways. Already, algorithms are determining pricing, security risk, creditworthiness, health, a person's intent, and many other things that shape the human experience.
Why AI competence will matter
One does not need to understand the details of electricity to turn on a light. Yet, it's pretty obvious that faulty wiring should be replaced by a licensed electrician. Like electricity, AI can be both beneficial and dangerous.
"Competence is a very intuitive concept in all domains and somehow it has not translated to AI and the operation of AI," said Nicolas Economou, chairman and CEO of AI-enabled eDiscovery solution H5. "The higher the stakes and the more other people are at risk beyond yourself, the more demanding standards of competence should be. In my domain, anyone can say I am competent to effectively exercise this scientific domain in the legal system and society should trust me."
Generally speaking, the concept of competence extends past licensed and certified professionals to novices who need to be educated about basic safety issues. For the latter group, "education" tends to take the form of written instructions and warnings so the person does not harm themselves or others.
"People who are scientifically trained in AI understand its limitations very well, what a blunt instrument it is and how it can be impacted by a range of things," said Economou. "In the [legal field], there has been so much carpet bombing of baseless claims about the magic of AI that what happens is you have a lot of lawyers and judges that simply believe it works. If you see something the AI tells you, it's correct."
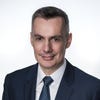
Nicolas Economou
Right now, there is no AI body that is equivalent to the American Medical Association or American Bar Association that can attest to a person's level of competence. Since the use of AI transcends any particular industry, it is likely that consortium of AI experts will sow the seeds of what an AI competence certification program should look like and then professional and vocational organizations will interact with that expert body to determine how the concept of AI competence should be applied to their membership. Already, some of the big judicial education centers are asking what they should know, Economou said.
In the meantime, law makers, regulators and the courts may decide that some type of instruction or warning is necessary for novices so they can operate AI or AI-enabled systems safely. If they do, the instructions or warnings would have to be appropriate for the system itself whether it's an internal enterprise system, consumer electronics product or an autonomous weapon.
"Competence is two things: the skills and experience a person must have to operate any kind of technology such that a certain goal can be met and evidence that the person actually has the skills they claim to have or should have to operate the technology such that the goal can be achieved," said Economou. "It's very surprising that nobody thinks of that when it comes to AI."
Competence is part of a larger trust picture
Competence is one of four concepts that are necessary to enable trustworthy AI. The other three are transparency, effectiveness and accountability.
Transparency (aka explainability) is probably the most-discussed topic given the current state of privacy concerns and related laws. However, more fundamentally, without transparency humans can't understand how an AI system (usually a deep learning system) works. While the majority of individuals won't be expected to understand the technical details of AI, they may well be asked by a business leader or an auditor why the system decided to take a particular action or the reasoning that resulted in a certain recommendation.
Effectiveness means that the AI system is capable of performing its intended function (solving a target problem). For example, a criminal sentencing algorithm must be capable of determining a reasonable jail sentence in a fair manner. However, skewed results can occur when the data is biased, the algorithm is biased or both are biased.
The fourth concept is accountability. Of the four concepts, accountability is most closely related to competence because it involves people. When an AI system malfunctions for whatever reason, it is likely that an aggrieved party will want to hold a person and/or other entity responsible.
Like multichannel and omnichannel marketing attribution, accountability will be difficult to determine because there may be several factors involved and the relative contribution of the individual factors that led to a result may be difficult to determine.
Bottom line
The race to implement AI systems should be tempered with prudent risk management to minimize the possibility of unintended outcomes. Competence is but one factor to consider. However, it's an important one as the number of AI use cases continues to expand and enterprises find themselves under pressure to manage the risks.
For more on AI and machine learning in the enterprise check out these recent articles.
Four Ways AI Can Augment Human Capabilities
Banks Ramp Up Machine Learning, Work Through Data Challenges
About the Author
You May Also Like