Financial Services Explores GenAI in Interesting Ways
Usually a laggard, financial services institutions are now experimenting with generative AI across all aspects of their business.
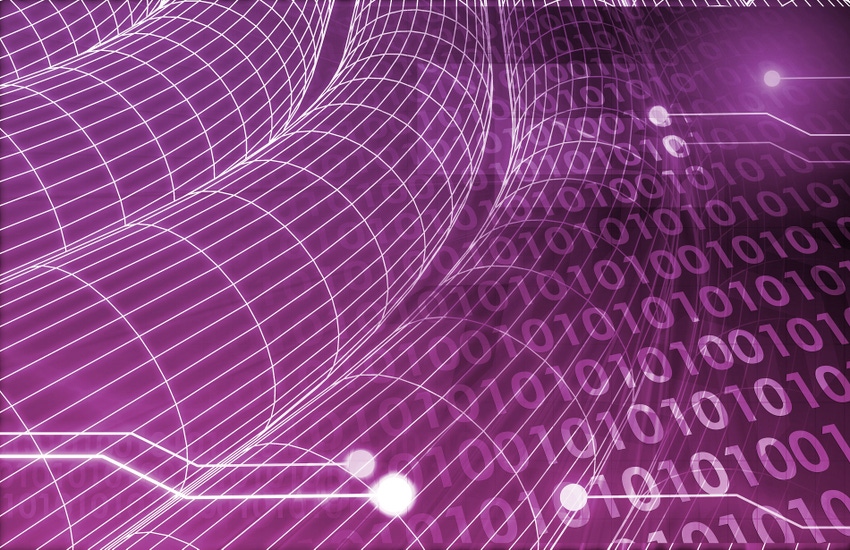
A surprising finding in our recent Generative AI Radar – North America survey was that the highly regulated, risk-averse financial services business was among the leading adopters of generative AI: Thirty-two percent of participating financial services organizations had either implemented or were currently implementing, generative AI solutions, while 23% had established use cases that created business value. Usually slow to try new technologies, financial services companies seem to be making an exception with generative AI in anticipation of its impact throughout their business by enhancing user experience, improving content generation and creativity, increasing operational efficiency and automation, and streamlining product development and design.
Specifically, financial services and insurance companies in North America that participated in the survey saw potential for using generative AI to produce and personalize policy documents and customer communications, extract information from financial documents, generate synthetic data to train machine learning models to detect fraud, and assist in regulatory compliance, to name a few. But in addition to working on the usual suspects, financial services companies around the world are deploying generative AI in unexpected ways.
Here are a few examples:
For assessing creditworthiness and risk: Banks can use generative AI in core functions, such as credit scoring and risk management. In place of traditional scoring methods, they can use machine learning and generative AI to analyze vast and varied data from multiple sources to create a more holistic evaluation of a borrower’s creditworthiness. Similarly, they can train generative AI on historical data to identify financial and other risks before they blow up.
For generating financial advice: Financial and investment advisory firms can train generative AI on proprietary customer data -- financial status and goals, risk profile, spending behavior etc. -- to generate recommendations on budgeting, trading and investing, managing risk etc. They can combine this with their human expertise to offer comprehensive and highly personalized advice to customers.
An example here is JP Morgan, which has developed a financial advisory tool called IndexGPT.
For product pricing and explanation: In the case of products where they have some pricing flexibility, financial services companies can use generative AI to understand customers’ willingness to pay and thereby charge the optimal price. Another interesting application is to use generative AI to compose easy to understand product descriptions and comparisons to help customers make the right selection.
For improving financial behaviors: Why do people persist with injudicious financial behaviors, ignoring rational counsel? Well, it appears that emotions have a key role to play in how people react to advice. Generative AI could step in here to correct customers’ financial behaviors by appealing to their emotions. Simple applications of this kind already exist, with chatbots and apps using humor and encouragement to promote certain behaviors. These interactions can be improved by using generative AI to compose more detailed and meaningful responses. It is also possible that generative AI may assist human advisors in more involved interventions by gathering customer inputs and highlighting the emotional triggers that can be used to modify their behaviors.
Mixed Bag of Opportunity
According to one research report, the generative AI in financial services market will multiply nearly tenfold between 2023 and 2032 -- from $1,186 million to $11,220 million at a CAGR of 28.36%. While the industry could potentially benefit greatly from this technology, it must also be cognizant of the risks. Currently, generative AI’s value mainly comes from its ability to create content based on large datasets containing text, code, images, and videos, at speed. For a knowledge, communication, and documentation-intensive business such as financial services, GenAI’s natural language capabilities are particularly relevant. Banks and financial institutions can use the technology to summarize large documents, offer customer support, or draft new content at much lower costs than with manual effort. Not just that, they can also use GenAI tools to amplify their employees’ performance. Besides productivity and cost efficiency, the list of GenAI benefits includes simplified operations, better risk management and fraud detection, improvement in customers’ financial literacy and (financial) health, enhanced user experience, and faster, more accurate decisions.
At the same time, the use of generative AI brings certain concerns and risks for financial institutions. Data challenges rank right at the top: If the dataset being used by the generative AI model is not of good quality, the outcome can have all types of flaws, including inaccuracy and bias (causing discriminatory credit decisions, for example); also generative AI algorithms can make mistakes, spread misinformation, and even hallucinate on occasion. Financial services organizations must also ensure ethical data usage, with full respect for security, privacy, confidentiality, and intellectual property rights by retaining a human in the loop to supervise the working of generative AI models. Also, as generative AI regulation evolves, very likely with regional differences, the highly regulated financial sector will face an even heavier compliance burden. The industry will also need to build the right talent by upskilling existing employees or hiring gen AI specialists; in addition, every employee will need to be trained on how to use the tools.
Give it Time
Financial services organizations are upbeat about the potential of generative AI to transform their business. While generative AI is growing at a rapid pace, its results may take time to show, since most companies will spend the next few years testing their models or piloting simple use cases, before progressing to large-scale initiatives.
About the Author(s)
You May Also Like