How AI Can Make Data Centers More Efficient and Decarbonize
As data centers struggle to tackle energy efficiency and decarbonize, AI has emerged as a powerful solution. Let’s look at the technology -- and where it falls short.
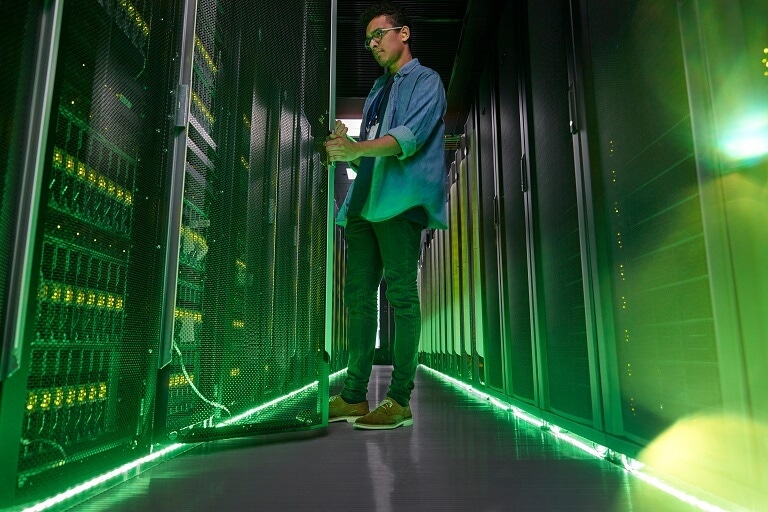
Data centers and other cloud computing operations are now thought to constitute up to 1% of global power use. The carbon expended in running these massive server farms -- and especially, in cooling them -- is far from insignificant. Some 50% of electricity use is thought to relate to basic operational costs and up to 40% is attributable to cooling costs.
Data centers are searching high and low for solutions, from leveraging more renewable energy to plopping data centers under the sea in order to save on cooling costs.
Some of the most parsimonious and practical solutions involve implementing artificial intelligence to locate and correct inefficiencies. A report by Gartner estimates that AI will be operational in half of all data centers in the next two years. A 2019 report by IDC suggests that may have already happened. Workloads are set to increase 20% year-on-year, so this is an urgent problem.
Ian Clatworthy, director of data platform product marketing at Hitachi Vantara, and Eric Swartz, VP of engineering for DataBank, speak about the possibilities and limitations of AI solutions in data centers.
Collecting the Proper Data
In order to create and calibrate useful AI instruments, data centers must collect and input the proper data. This has proven challenging because certain types of data that have not been historically useful in day-to-day operations have simply been ignored. Some may be collected but unused. And some is not collected at all, meaning that operators have to start from scratch or extrapolate from existing data.
Necessary hardware data includes: the available storage, the ease of access, the number of machines running at a given time, and the machines to which traffic is directed under any given circumstance. Data relating to energy expended on powering machines and cooling is also essential, as is related data on environmental conditions inside and outside of the center.
“In order to be able to build a proper machine learning AI system, you would need all of that to really dial in the efficiencies. All of that matters,” says Swartz. “Every one of those data points can skew the other.”
AI can in fact be useful in collecting this information in the first place. Data mining can extract useful data buried in seemingly unrelated statistics given the correct instructions. When the proper data is arrayed, according to Clatworthy, it can “actually present information in a way that means something.”
How to Leverage AI to Create Efficiencies
Power use by servers is a main target for AI intervention. Servers that are not in use are left running and incoming traffic is inefficiently distributed across available equipment. Scheduling control engines can use deep learning to direct traffic appropriately. It can be distributed across available machines in a manner that makes optimal use of their capabilities but does not overload them.
And then unused machines can be powered down until they are needed. Better yet, says Clatworthy, “We can turn the CPU down. By turning things down, you use less power.” Powering machines on and off, he reasons, is inefficient too.
Traffic patterns can be anticipated, thus enabling more frugal usage of equipment. Power usage effectiveness (PUE) is thus improved. AI can help in scaling these processes as workloads increase.
Further efficiencies can be created by predictive maintenance. “By understanding historical data on component problems or maintenance schedules, and tying that into budget allocations, organizations can use AI to provide predictive models,” Clatworthy says.
Ian Clatworthy, Hitachi Vantara
By leveraging data to ascertain when outages are likely to occur, appropriate backups can be established more easily. Patching and upgrading, which are onerous and labor intensive, can be automated to an extent as well. And failing machines can be replaced or repaired before they cause interruptions in service.
Management of power sources themselves can benefit from AI as well. By determining when renewable sources are most available -- windy days for wind power, sunny days for solar -- data centers can target when they pull from these sources and when they resort to less desirable sources of electricity derived from fossil fuels. Waste heat can be redirected and used either within the center itself or by surrounding facilities.
“You can't just always be on renewable energy,” claims Swartz. “By using AI to figure out when the best times are to use it, you get the best of both worlds.”
There are cost savings there as well. “Even 1% [of power usage] can mean hundreds of thousands of dollars in energy,” he adds. “To dial it into the most efficient operating parameter would be very beneficial.”
Cooling systems are another target of AI efficiency programs. Like power, they have in the past been constant. That is, they were not adjusted according to changing parameters but instead ran at steady rates determined by vague estimates of need.
Cooling is very expensive -- both financially and in terms of carbon emissions -- and even minor tweaks to cooling systems can amount to substantial savings. Thermal management must take into account such factors as ambient temperature, weather, the heat generated by active machines at any given time, the materials of which the building is constructed, and the current HVAC systems in place.
AI can direct cooling activities to the systems that need it -- down to specific racks of machines -- and shut them down in areas that don’t. It can even factor in lag time, anticipating when certain sectors will be powered back up and directing cooling to them in advance.
How Digital Twinning Can Optimize Data Center Systems
Creating a digital twin, or virtual representation of the physical environment of a data center can help to model how its various components interact without risking disruption to the system itself. By inputting data regarding energy, temperature, traffic demands and weather, among other factors, AI architects can devise optimal conditions for data centers -- theoretically at least.
“We can simulate different cooling configurations,” offers Clatworthy as an example. “Whether that be Singapore, in Melbourne, in Europe, in the rain -- we can identify the most efficient cooling layouts based upon the location of equipment.”
Missing data -- and there’s always missing data -- will of course skew these digital models. But even a reasonable quantity of historical data can create realistic models of how data centers actually operate and use energy.
Digital twins are not self-sustaining, though. They require tuning by human observers, who can flag parameters that exceed what might be possible in the physical world. Thus, the models are refined over time.
Challenges to AI Deployment in Data Centers
Data scarcity represents the most vexing challenge to AI implementation in data centers. While some data is harvested for other purposes and is thus ready for input into AI systems, some data that is essential to optimize AI performance has until now been adrift in the digital ether. Some can be retroactively harvested from other sources. But other types require new methodologies -- meaning that there is no historical record. Data centers must start from square one.
For example, data centers have the manufacturer-specified power consumption of an out-of-the-box machine at their disposal. But the power consumption of machines as they age and their performance deteriorates may not be collected -- and is thus not available for entry into AI solutions. Intimate knowledge of the capabilities and vulnerabilities of each piece of equipment in use is imperative -- and often difficult to obtain.
As Swartz notes, multi-tenant data centers face another level of difficulty in collecting data, as they must abide by privacy agreements with their clients. “We have different types of customers with different needs and different levels of risk,” he imparts. “When you're trying to accommodate all of that, you are not able to typically be the ones who are living on the edge.”
Eric Swartz, DataBank
AI also requires new and complex systems and equipment to support its implementation -- the so-called AI tax. It is not cheap upfront but cost savings down the line appear to be reliable. Still, getting the system up and running is no small task -- data must be gathered, processed, input, and then re-analyzed.
Making sure that data centers can communicate with each other in a sustainable way is a further challenge. “We're looking at how to use AI software to move data from data centers with no impact at all to the customer,” says Clatworthy. This presents any number of obstacles when renewables are factored in. “The sun's going down here. This means we're not going to use renewables to move this data set.”
Even as their sophistication grows, and they navigate such decisions, AI systems are still no match for human reasoning in some situations.
“AI does not yet have the ability to make complex strategic decisions in a timely manner,” Clatworthy observes. “I want it to tell me what my capacity is going to be long term, tell me what needs to be upgraded. I'm going to focus my team on unforeseen anomalies.”
As AI becomes ever-more integral to data center operations, its human handlers will have to adjust their responsibilities accordingly.
What to Read Next:
What Are Scope 3 Emissions and How Can Data Centers Address Them?
About the Author(s)
You May Also Like